top of page
Accurate and robust localization is critical for autonomous driving. Even the state-of-the-art multi-sensor fusion platform (GNSS/INS/LiDAR/HD Map) could be severely challenged in the highly urbanized areas with tall buildings and dense traffic. Major challenges come from two parts: 1) excessive sensor outliers, such as GNSS outliers in dense urban; 2) varying sensor error models as errors are not always subject to Gaussian distributions.
The existing datasets for autonomous driving don’t necessarily demonstrate these challenges for localization. Therefore, we present a challenging multi-sensor integrated localization dataset. We traversed various scenarios with different degrees of urbanization in both Hong Kong (by Hong Kong Polytechnic University, Intelligent Positioning and Navigation lab) and California (University of California, Berkeley, the MSC lab). The measurements came from multiple sensors including a global navigation satellite system (GNSS), an inertial navigation system (INS), camera and light detection and ranging (LiDAR). The dataset is available online (please see the downloads).

UrbanLoco Dataset
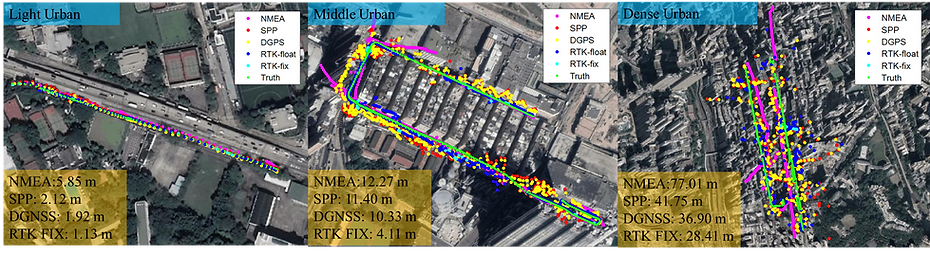
GNSS positioning performance in typical urban canyons of Hong Kong
Sample use of the dataset
Citation:
To see more details, please find the conference paper here. This work is finished by a team from the IPN Lab at the Hong Kong Polytechnic University and the MSC Lab at the University of California, Berkeley.
Weisong Wen, Yiyang Zhou, Guohao Zhang, Saman Fahandezh-Saadi, Xiwei Bai, Wei Zhan, Masayoshi Tomizuka, and Li-Ta Hsu, UrbanLoco: A Full Sensor Suite Dataset for Mapping and Localization in Urban Scenes, ICRA 2020, Paris, France.
License
This work is licensed under a Creative Commons Attribution-NonCommercial-ShareAlike 4.0 International License and is provided for non-commercial but academic use. If you are interested in using this dataset for commercial purposes, please contact us.
bottom of page